Article 2 from the Series: Winning in the data economy
Topics covered:
1. Conceptual business case
2. The benefits
3. The costs and opportunity costs
4. Comparing and measuring
5. Conclusion
Remember the days when every capital project needed a business case and they all claimed they’d save headcount in the contact centre? I’m pretty sure when all those business cases got stacked together the contact centre was running on tumbleweeds! Those business case could be modelled by project and capitalizable costs and compared to the ultimate people, tech and other OPEX savings (or value creation) resulting in an ROI at 3 – 7 years.
The AI business case still has the cost and benefit sides of the equation of course, but there are five key things that make it more complex:
- The output is not AI only (it’s never just an AI project) – it’s got all the usual software and engineering costs loaded too. Sometimes the business case may actually be achieved by process oriented change rather than the AI aspect of the project.
- The accuracy and impact of a model may be completely unknown until models are actually built; which may not be able to be done without the requisite data (often a dependency on vendors or other business units).
- The scarcity and specificity of the right people (data engineers, analysts and data scientists and so on) will impact cost and quality.
- The pace of change that results from AI means that the benefit horizon needs ongoing investment and maintenance.
- If you are going about it the right way the business case could be compound and the benefits implausible or unbelievable.
Even though the framing of the business case might be consistent it’s a conundrum for many customers as to how to build a business case on these shifting sands.
Throughout this blog I’m going to refer to a common use case in the MVNO space ‘Developing a personalised Customer Experience’ as an example.
The Conceptual business case
Firstly, with an AI project ask yourself why? Not just why am I doing it; but more importantly, why does it need to be an AI project. AI projects are unpredictable by nature so the first step of the business case should really be about making sure that what you’re doing really needs AI to be applied (and yes as the CEO of an AI business I realise the irony of saying this!!).
Take the ‘Personalised Customer Experience’ as an example – there’s a lot of personalisation that could be applied with simplistic marketing tools and a few business rules. One simple gotcha is the need for enough data – if you’re not seeing a pattern literally thousands of times it’s pretty unlikely that AI is going to do a better job than those business rules.
The second question is who benefits? This is where AI projects really diverge from normal tech projects. If you tackle an AI project the wrong way then you are talking about who ultimately benefits but if you take a broader view and you’re really smart about the use of data there are usually multiple beneficiaries – the concept of data products comes from this insight. Back to the personalisation use case – the same data used for personalisation should be used for churn prediction, customer services, it could be an insight used for marketing strategy, it could even be monetizable. In other words, the beneficiary isn’t just the project initiator.
The third question is about making the choice about build vs buy and whether build means build everything or there is tooling that can support you. This is pretty similar to a traditional technical project but in my experience the decision to build can be materially underestimated. I can think of one brand that I met some time ago that thought they were buying (as opposed to building). They invested in Snowflake but they quickly realised that this required technical expertise that they did not have in house and required data transfer costs to be incurred which they hadn’t expected. There’s no one answer but in my experience there’s hidden cost in the tooling, cloud resources and a huge misunderstanding of the specialisms required to build and operate high accuracy AI models especially when you want them to have high degrees of explainability and actionability too.
Anecdotally, I’ve seen scaled personalisation use cases deployed for CVM return an ROI within 6-9 months of deployment; the alternative is a team of 5 people and a time to value of +12 months (more likely 24 – 36 months).
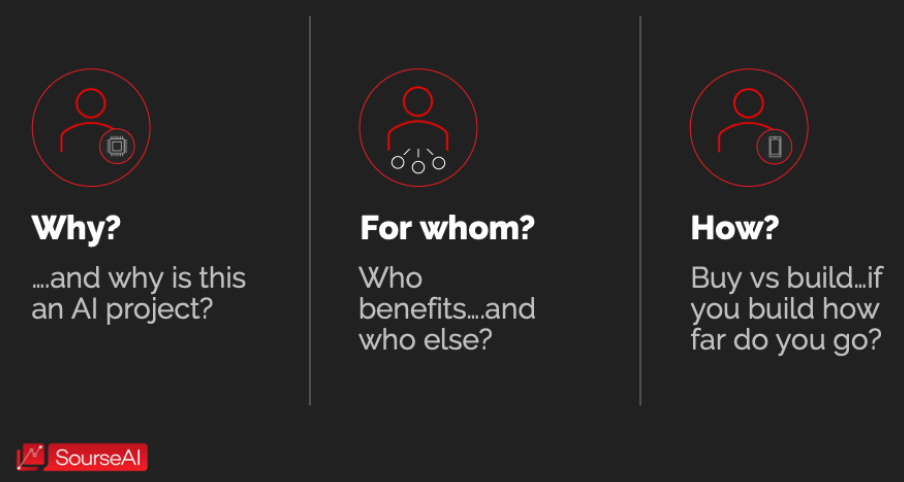
The benefits
You wouldn’t be the first (or last) project whose driving force was ‘My board / MD / CEO told me to have an AI strategy’ but that’s certainly not a reason that creates ROI – in fact there’s probably some correlation that’s the opposite.
You’ll know what your benefits are but it’s worth thinking about the benefit of:
- Doing the same things better
- Doing new things
- Making existing processes obsolete
Going back to the example ‘Personalisation of the Customer Experience’ you could for example regard this as better marketing than ‘Hi *F-name*’ but you might also consider that you are entirely flattening complex, multistep customer journeys built in marketing automation platforms or eradicating entire processes that you need to support.
If you restrict your benefit to ‘doing the same things better’ the business case might be great but when you think toward making processes obsolete the business case compounds because the same costs can be applied to a bigger and more complex outcomes.
McKinsey’s research puts ‘The Personalisation Effect’ at being able to uplift APRU by 10% and being able to contribute to a 20-30% increase in Customer Satisfaction.
The Costs and Opportunity Costs
The hidden cost of AI projects fall into three broad categories):
1. Culture change: If you’re aiming at big problems then make sure that you account fully for culture, leadership and data fluency. You need to create an environment where you value learning over management, insights over opinion (and seniority) and iteration over perfection.
2. Specialism: It takes a group of specialists to do this really, really well – Data Analysts, Analytics Engineers, ML Engineers, Data Scientists and data literate business people in collaboration. These aren’t the same skills and you’ll be doing any of these specialists a disservice to ask them to be a bridge. It’s a little like expecting your local panel beater to repair your roof – they’re all panels, right?
3. Tooling: I’ve touched on this point already, but your AI project needs tools to organise, structure, ingest, interpolate, infill, transfer, QA, translate, store, build models, train models, receive outputs, orchestrate, schedule and experiment (etc!). These tools are rapidly evolving – being an expert is one thing and staying expert well that’s a totally different game. Tooling is always underestimated along with the effort to establish the process even before the data science or AI part gets started.
4. Unpredictability and failure: The DNA of a data driven business is built on experimentation, curiosity and the embracing of learning which sometimes is failure. In extreme cases, the cost of failure is an unknown timeline to value but in some cases the reality is that the expected benefits are not unlocked. In many cases the benefit and its quantum may not be known until the data is explored and the outcome is achieved.
This is one of my favourite charts (credit to McKinsey) and it makes the point about hidden costs perfectly. If you’ve already been investing in tools, specialists and culture you’re on the steep part of this curve and reaping the rewards. If you haven’t invested already then catching up requires ‘buying’ to catch up to early adopters.
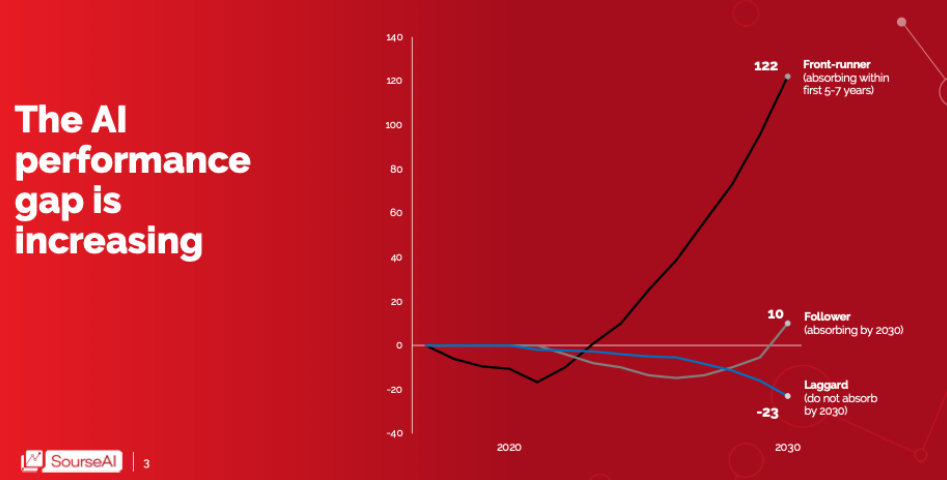
There’s one last aspect to the costings side and that is the cost to invest in ongoing challenger models, measurement and improvement. This is an investment that is ongoing – it’s not a project that is delivered and handed to a BAU team; it’s a new capability that needs to be refined and improved.
Comparing and measuring
I’ll cover this in more detail in the third party of the series – it’s a big topic. The most important thing however is to measure impact through the connected tissue of your business. That’s the way you bring together intellectual curiosity and scientific method and hold them to account in continuously evaluating your approach. (More on this in January!)
In conclusion
MVNO’s like it or not you are operating in the data economy. Going beyond a tech brand to becoming a data brand needs a different business case – one that looks at the broader opportunities AND the hidden costs. The business case is likely to be less predictable, more interconnected across your business and have a less certain payback. I hope you’ve got some useful ideas about the data foundations you need to win in the modern data economy – we help telcos build their data strategy and adapt to their AI driven future. Please be in touch if you’d like some advice.
What’s next in this Series: Measuring, learning and keeping up with AI
I’ll be writing a follow up article in January building on this blog. Feel free to be in touch if there’s anything specific that you’d like to know about measuring the impact of AI.

Guest Blogs are written by carefully selected Experts. If you also want to create a Guest blog then Contact us.